Innovation
The Human Centered Big Data project produced new methods for understanding, visualizing and explaining the reasoning process of “black box” AI algorithms, resulting in more trustworthy and interpretable algorithms. Along the way, we developed novel approaches for a number of AI-assisted tasks including image interpretation, image search, context-aware text search. Results of the project included new algorithms for quickly searching through large image databases, methods for inferring how neural networks organize input data, and techniques for explaining that organization in human-understandable forms, and novel techniques for training neural networks to operate on human-interpretable text rules.
Background
Society has now moved into a present where AI lives in our pockets, helps us avoid car accidents, evaluates our suitability for insurance plans and loans, manages our investments, helps doctors diagnose disease symptoms and recovers information from videos and images to help law enforcement and our military forces. Yet in applied contexts, especially in those where decisions have personal, financial, safety, and security ramifications, the present art in AI systems that use statistical machine learning leaves an important question unanswered: when a machine makes a recommendation, can a human who is accountable for the decision trust it? For example, intelligence analysts throughout the government employ a wide variety of machine learning, pattern recognition, and multivariate statistical modeling approaches to assist human analysts in the retrieval, organization, analysis and interpretation of high-throughput data. Many of the machine learning approaches that have proven most effective in these tasks lack transparency. The result is an algorithm that may, for example, be very effective at a specific analysis task, but that is unable to “explain” what factors led to its conclusions. This lack of transparency is not only detrimental to human trust, but can, in fact, be dangerous in that fallacious or brittle lines of reasoning by the algorithm are not exposed.
Commercial Goal
The project resulted in 8 new jobs, 4 jobs retained, an executed license agreement, $9.14M in new federal funding awarded to the investigators or including research ideas spawned in execution of this work, and the founding of a new company with headquarters in Fairborn, OH. In execution of the project, the researchers built enduring collaborations with researchers in government (AFRL) and industry (datascience.com, NCR Research Laboratories, and others).
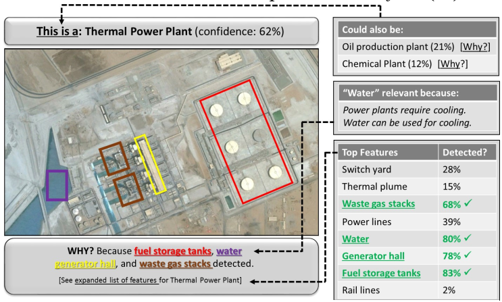