Innovation
- Adaptable experimental design in Flight Simulator: Researchers can create different scenarios in the application (PiTA: Pilot Trust in Autonomy) with different routes, goals, and biometric sensors. They can customize or create new data fields.
- Adaptable experimental analysis: Data exploration and visualization analysis tool (Visionary) allows analysts to focus on any experimental variable(s). Database architecture and filtering features of Visionary greatly reduces data cleanup and provides quick look and deep dive analysis.
- Research into flight control input patterns: Demonstrated predictive power of classification algorithms towards identifying the skill level of operators using only controller input. This research was published in the Institute of Electrical and Electronics Engineers International Conference on Machine Learning.
Objective
Develop autonomy algorithms to improve the performance of HMTs. The major tasks of the project, which drive toward the goals, were delineated as follows:
- Conduct a literature search of previous research in this area and analysis of business opportunities.
- Develop software to collect time-aligned data from biometric sensors and human control input in a virtual environment (ultimately a flight simulator).
- Design experiments, collect data, and develop predictive algorithms around performance.
- Design and test methods of sliding-scale augmentation in a virtual gaming (ultimately became a flight simulator) environment.
Background
Problem: One-size fits all design of HMI systems and augmentation strategies is not optimized for individual variability, experience, trust in the system, and proficiency.
Opportunity: Current industry methods for augmenting human machine teaming are focused on reducing workload independent of the operator state. A challenging opportunity is to model workload as a function of physiological indicators and to design augmentation solutions that adapt the level of autonomy based on the human operator’s skill level, workload, and performance.
Commercial Goal
According to Dr. Reiter’s final brief, the project generated $7.725M in follow-on funding from a broad customer base including the Office of the Secretary of Defense, Defense Advanced Research Projects Agency and United States Special Operations Command. The OFRN model that joins universities, the federal government and industry partners provides unique opportunities and networks for R&D. Five (5) new non-profit jobs created. Twelve R&D jobs were retained. The research focus of this project produced one Master’s degree.
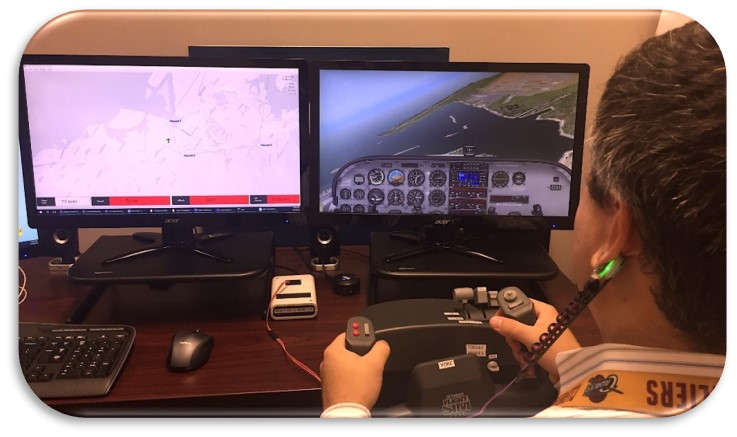